The Automated Future at CMU
While beakers and Bunsen burners will likely never go away, chemists in the 21st century are increasingly making room on their lab benches for new technology that can aid their research. From advanced computer programs that can learn to design theoretical molecules on their own to robots that can run basic chemical tests and reactions precisely and quickly, the field of chemistry is embracing machine learning and automation.
A growing group of researchers in the Department of Chemistry has begun harnessing these tools to improve and advance their work.
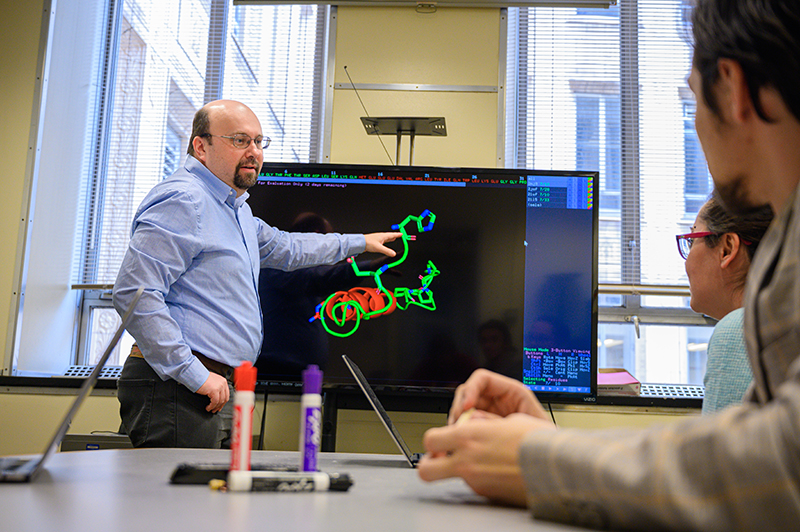
Olexandr Isayev explains a theoretical molecule designed by his lab.
Olexandr Isayev
“A focus of our lab is connecting chemical sciences with artificial intelligence (AI) and machine learning,” Assistant Professor Olexandr Isayev explained. “I believe that those technologies could help us solve some of the fundamental chemical challenges.”
Isayev, who came to Carnegie Mellon in January, has been interested in the idea of blending computer science with chemistry ever since he came to a fork in the path of his academic studies. “I chose chemistry but I always had this idea about computers and AI in the back of my mind. Now I have the chance to reconcile it and use both technologies.”
One major focus of his burgeoning lab is designing new molecules that could be used for novel drugs or materials. Typically, in chemistry, Isayev noted, serendipity has been behind many molecular discoveries. But simply mixing different chemicals together and seeing what happens can be time-consuming and expensive.
“We want to use AI to transform discovery of new molecules and materials,” Isayev said. To that end, a Ph.D. student in his lab, Maria Popova, has designed an algorithm inspired by the game-playing program AlphaGo.
Instead of playing Go, however, this algorithm plays a game with itself in which it designs molecules and then critiques them to see whether they’re feasible and useful. Over time, the algorithm employs machine learning to improve its designs by learning from its mistakes and successes.
“This algorithm can eventually dream up molecules that have been predicted to have desired biological properties,” Isayev said.
Isayev’s team has applied this AI-driven work toward aiding the search for potential drugs for COVID-19. Working with the Pittsburgh Supercomputing Center, the researchers were able to add to the libraries of small molecules that scientists search through when looking for new therapeutics.
Another focus of his lab is using machine learning and AI to improve research into quantum mechanics. For example, the field’s landmark Schrödinger’s equation cannot be solved analytically; therefore, it usually requires a lot of computing power to solve it numerically. However, with deep learning potentials that Isayev’s lab is developing, complex molecular dynamics simulations about how molecules are structured and react with each other can be calculated with most any computer. For Isayev, this “democratization” of quantum computational chemistry is an important goal.
“Not everyone has access to supercomputers,” Isayev noted. “So people who wouldn’t necessarily have the resources can do it on a laptop.
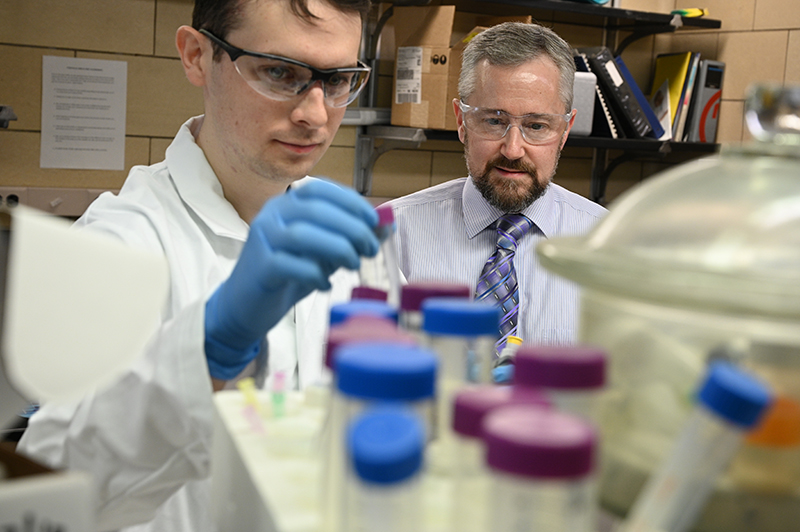
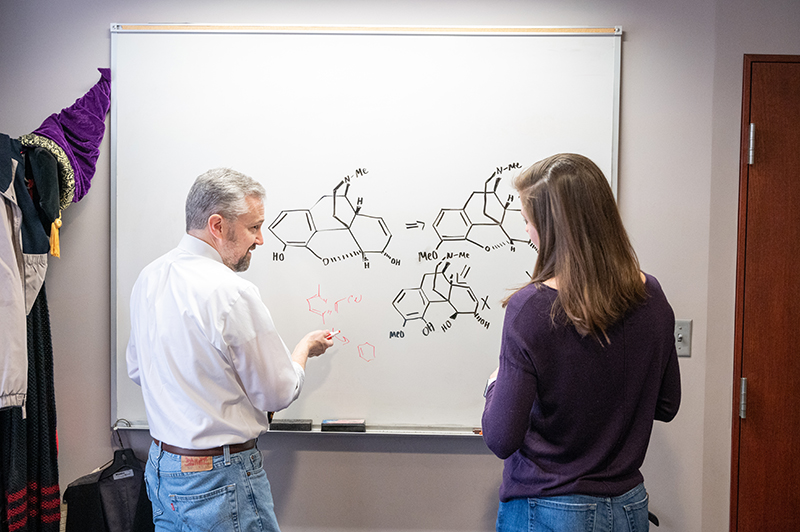
Upper and lower images: Bruce Armitage works with members of his lab on designing and synthesizing organic molecules.
Bruce Armitage
DNA and RNA are made up of thousands or even millions of molecules linked together, forming and expressing the genetic sequences that define life. Yet each of those many sequences also represents an opportunity for molecules to bind to the DNA or RNA and change how certain genes are expressed.
Since many human diseases have roots in our genes, interfering with their expression provides opportunities to study how the diseases work and even create potential therapies for them. This genetic realm of study is a focus of the lab of Professor of Chemistry Bruce Armitage.
“How can you synthesize and screen all those different possibilities in a reasonable amount of time? The answer is through automation.”
While the sheer length of human DNA and RNA sequences means many opportunities for different molecules to bind to different genetic regions, it also means thousands of different possibilities that need to be tested.
“So how can you synthesize and screen all those different possibilities in a reasonable amount of time?” Armitage asks. “The answer is through automation.”
Instead of having a researcher pipette ingredients into hundreds of test tubes to synthesize batches of a molecule, Armitage’s lab has been using robots to accurately automate that process for the past decade.
“Not only does it make that process faster, but it makes the results more reproducible,” Armitage said.
More recently, Armitage’s group has been moving into not only creating molecules through automation but also testing them. Armitage and other Carnegie Mellon researchers have been working with Mellon College of Science Dean Rebecca W. Doerge and the Emerald Cloud Lab, a startup founded by CMU alumni that does automated laboratory testing, to plan a similar advanced facility at CMU’s Pittsburgh campus.
Armitage sees great potential in automated molecular synthesis and testing in developing potential molecules and in helping scientists fundamentally understand how the human genome works and what its individual components mean for our health and lives.
“It’s going beyond the sequence of the human genome to what that information really means in terms of how does a cell function, how does it live, how does it die,” Armitage said.
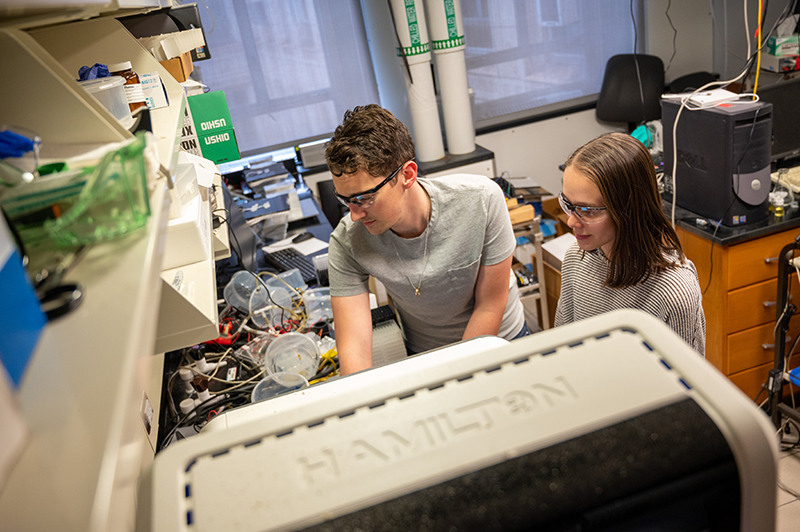
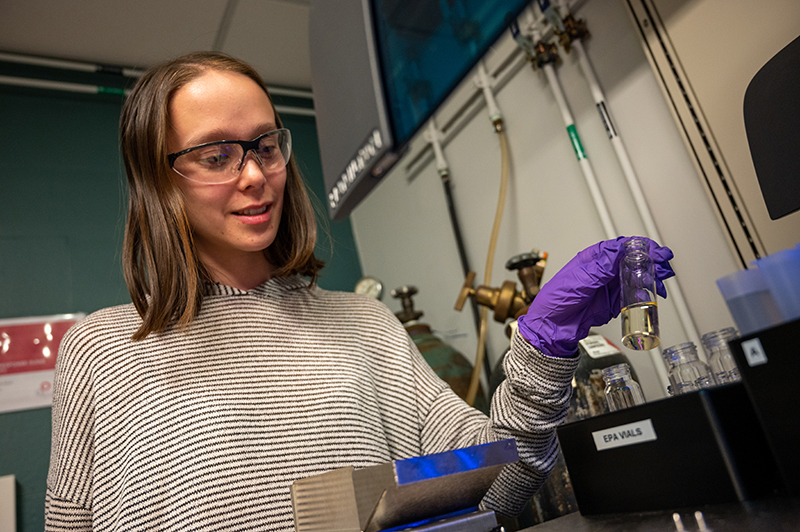
Upper and lower images: Members of the Bernhard lab mixing reagents.
Stefan Bernhard
Before Professor of Chemistry Stefan Bernhard embraced automation in his laboratory, he estimated he and his students designed about 2,000 total light-driven hydrogen evolution reactions for his research, of which he published around 500.
“This can now be done by a student in a week,” Bernhard noted.
Bernhard’s lab has been working to develop reactions that can convert light into chemical energy for the storage of solar energy. Using robotic devices, the researchers can rapidly and efficiently mix reagents together in many different shell vials to monitor and test them.
This automation helps not only with the physical processes in experimental chemistry but also with the analysis of results.
“If you make things easier to do in parallel, you get databases of results that you can then understand with computer-based, data science-driven modeling,” Bernhard explained.
However, while automation has made his research efforts much more efficient and productive, Bernhard said it also makes the upfront process of planning and designing experiments a much more expensive and challenging process.
“We have to think completely differently,” Bernhard explained.
To minimize costs, his lab seeks to build or repurpose as much experimental infrastructure as it can. This can result in some creative efforts, Bernhard said, such as hacking into a tire-pressure monitoring system to retool it for tracking the gas pressure of evolving hydrogen.
While hacking might not be something one would expect in a typical university chemistry laboratory, Bernhard said it’s not too difficult in MCS to find students for his lab that are up to the challenge.
“(Carnegie Mellon) undergrads have skill sets that undergraduates at many schools don’t have, which is a profound knowledge of programming, interfacing and data management,” Bernhard said.
♦ by Ben Panko