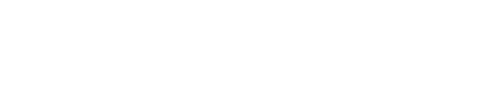
taking ai to the stars
From Feb. 23-25, the National Science Foundation AI Planning Institute for Data-Driven Discovery in Physics held “AI Super-Resolution Simulations: From Climate Change to Cosmology,” a hybrid conference showcasing artificial intelligence-assisted research across a myriad of disciplines.
Along with researchers from Carnegie Mellon University, the conference invited people from Japan, Iran and other nations to join. About 50 scientists were able to attend in person with 150 more attending online.
“The main idea was to just bring people in different disciplines together in a way that we hadn’t really done before to such an extent,” said Rupert Croft, professor of physics and member of the McWilliams Center for Cosmology, who helped organize the event. “CMU is interdisciplinary, and so we just noticed that people share a common language and now that common language is artificial intelligence. I think the most exciting thing was just to get these different communities together and everyone realized that they could actually understand each other.”
Because of this universal language, participants were able to understand each other’s research, even when it did not fall under their disciplines.
“There were people there who study distant galaxies, climate change, blood flow in the heart, racecar engines and urban planning, all linked by a common thread — the use of AI to speed up computer modeling of physics by factors of thousands,” said Croft.
Pedram Hassanzadeh, assistant professor of mechanical engineering and Earth, environmental, and planetary sciences at Rice University, attended and spoke at the conference. He appreciated the interdisciplinarity of the conference, and he is excited for the potential of AI in his own research.
“I think there is a lot for these disciplines to learn from each other on using AI for their specific applications,” said Hassanzadeh. “Super-resolution is critical for a broad range of applications in fluid mechanics and climate science. The approach can be used, for example, to better account for small-scale process in climate models for climate change projections, or to provide more details on wind power availability for renewable energy forecasting.”
CMU graduate students were also able to get involved. Yueying Ni, a physics Ph.D. candidate, gave an in-person talk on “Super-Resolution Cosmological Simulations,” where she talked about how she was able to use AI to discover where celestial bodies are based on the surrounding dark matter.
Croft was excited to have graduate students participating in the conference.
“We noticed that a lot of graduate student applicants have worked on AI in their undergraduate research and careers, and they want to continue using those techniques now,” said Croft. “Many of our keynote speakers were graduate students who were enthusiastic. There were even first-year graduate students who gave great talks, so that’s maybe a little bit different to usual conferences.”
Croft is hoping that these events can continue in the future with funding from the NSF. He hopes that other researchers can understand the potential of AI in their fields.
“We’d like to expand the range of what we’re doing, or we’d just like to hold events like this one,” said Croft. “We think that AI is going to become a part of physics in the way that experiments are, and pencil and paper are.”
■ Kirsten Heuring
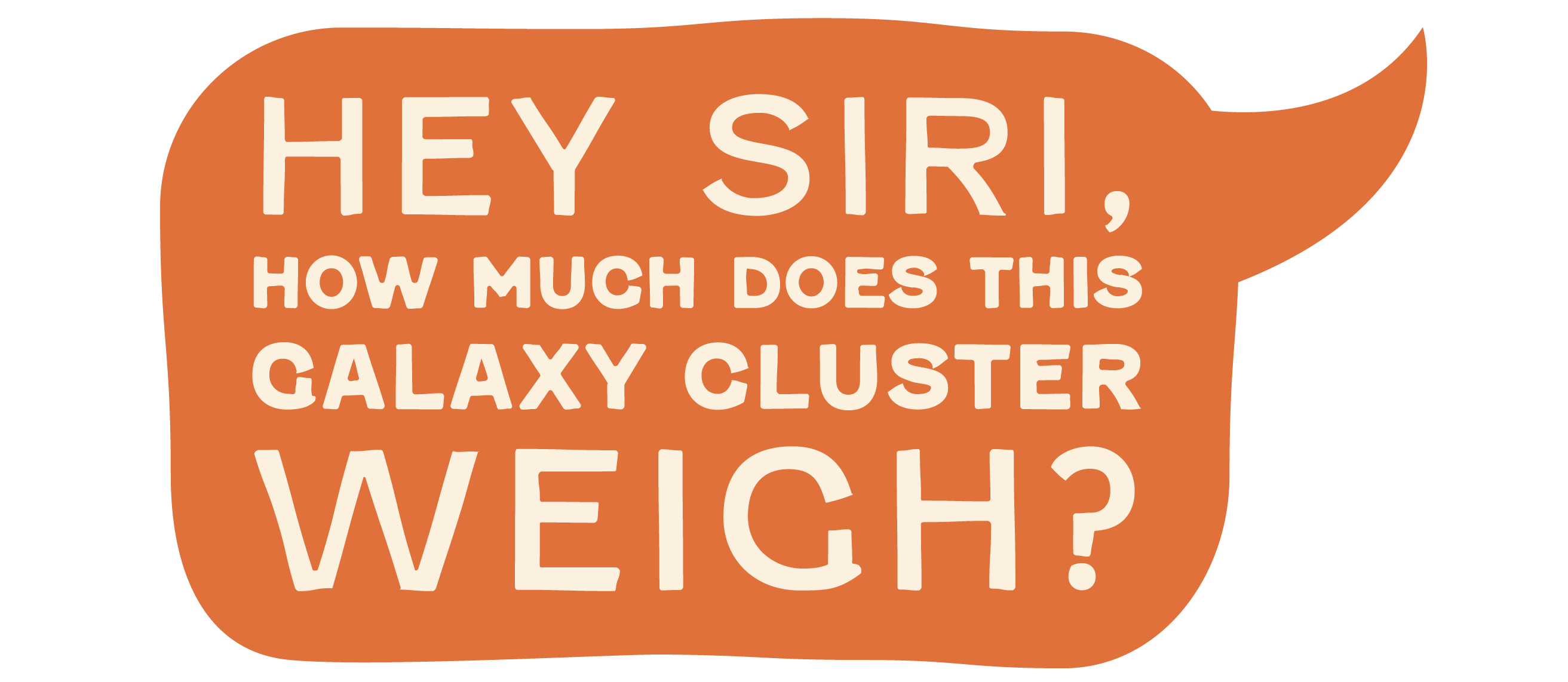
It’s been nearly a century since astronomer Fritz Zwicky first calculated the mass of the Coma Cluster, a dense collection of almost 1,000 galaxies located in the nearby universe. But estimating the mass of something so huge and so dense, not to mention 320 million light-years away, has its share of problems — then and now. Zwicky’s initial measurements, and the many made since, are plagued by sources of error that bias the mass higher or lower.
Now, using tools from machine learning, a team led by Carnegie Mellon University physicists has developed a deep-learning method that accurately estimates the mass of the Coma Cluster and effectively mitigates the sources of error.
“People have made mass estimates of the Coma Cluster for many, many years. But by showing that our machine learning methods are consistent with these previous mass estimates, we are building trust in these new, very powerful methods that are hot in the field of cosmology right now,” said Matthew Ho, a fifth-year graduate student in the Department of Physics’ McWilliams Center for Cosmology and a member of Carnegie Mellon’s National Science Foundation AI Planning Institute for Data-Driven Discovery in Physics.
Machine learning methods are used successfully in a variety of fields to find patterns in complex data, but they have only gained a foothold in cosmology research in the last decade. For some researchers in the field, these methods come with a major concern: since it is difficult to understand the inner workings of a complex machine learning model, can they be trusted to do what they are designed to do? Ho and his colleagues set out to address these reservations with their latest research, which was published in Nature Astronomy in July.
To calculate the mass of the Coma Cluster, Zwicky and others used a dynamical mass measurement, in which they studied the motion or velocity of objects orbiting in and around the cluster and then used their understanding of gravity to infer the cluster’s mass. But this measurement is susceptible to a variety of errors. Galaxy clusters exist as nodes in a huge web of matter distributed throughout the universe, and they are constantly colliding and merging with each other, which distorts the velocity profile of the constituent galaxies. And because astronomers are observing the cluster from a great distance, there are a lot of other things in between that can look and act like they are part of the galaxy cluster, which can bias the mass measurement. Recent research has made progress toward quantifying and accounting for the effect of these errors, but machine-learning-based methods offer an innovative data-driven approach, according to Ho.
“Our deep learning method learns from real data what are useful measurements and what are not,” Ho said, adding that their method eliminates errors from interloping galaxies (selection effects) and accounts for various galaxy shapes (physical effects). “The usage of these data-driven methods makes our predictions better and automated.”
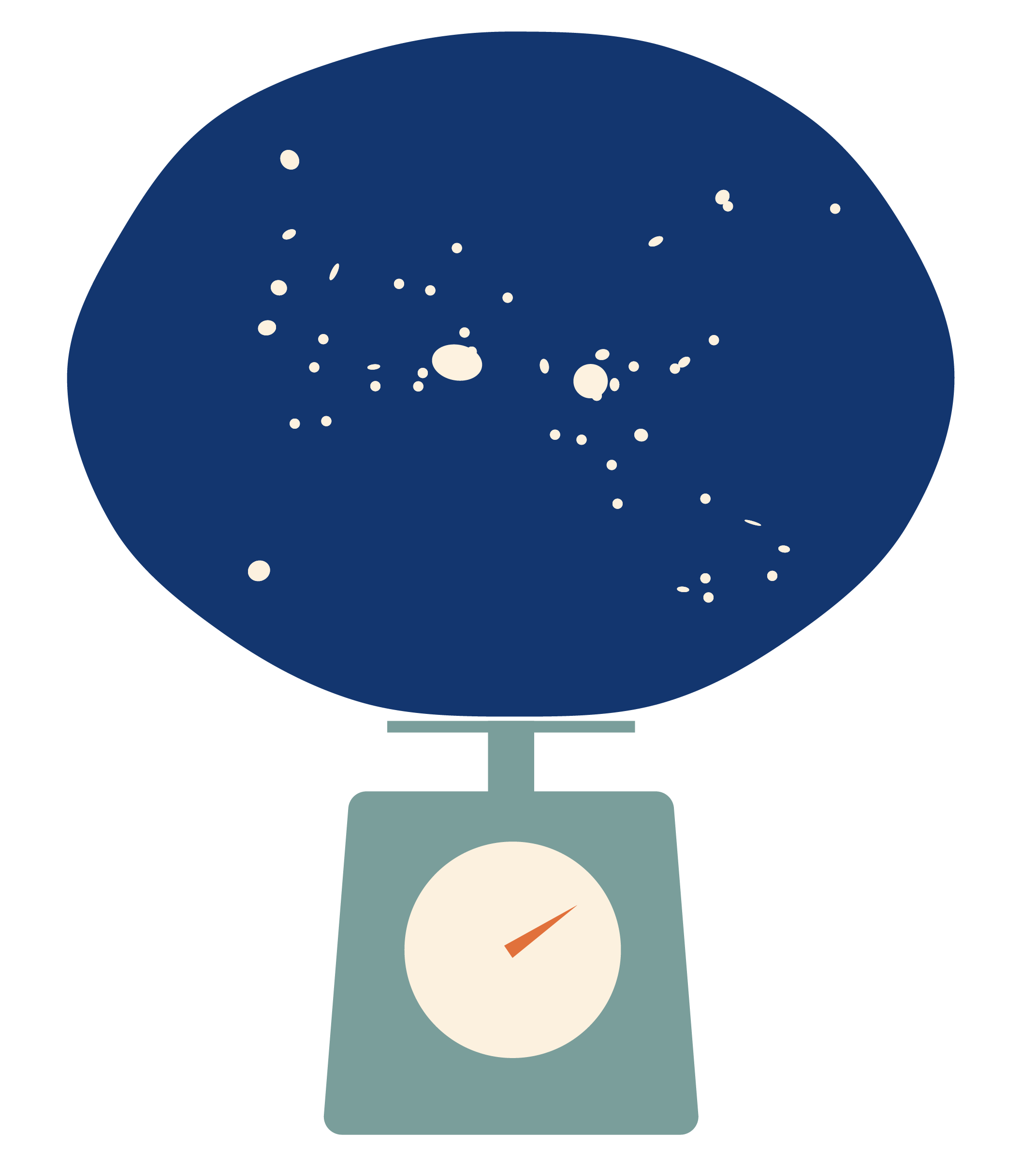
Ho and his colleagues developed their novel method by customizing a well-known machine learning tool called a convolutional neural network, which is a type of deep-learning algorithm used in image recognition. The researchers trained their model by feeding it data from cosmological simulations of the universe. The model learned by looking at the observable characteristics of thousands of galaxy clusters, whose mass is already known. After in-depth analysis of the model’s handling of the simulation data, Ho applied it to a real system — the Coma Cluster — whose true mass is not known. Ho’s method calculated a mass estimate that is consistent with most of the mass estimates made since the 1980s. This marks the first time this specific machine-learning methodology has been applied to an observational system.
“To build reliability of machine-learning models, it’s important to validate the model’s predictions on well-studied systems, like Coma,” Ho said. “We are currently undertaking a more rigorous, extensive check of our method. The promising results are a strong step toward applying our method on new, unstudied data.”
Models such as these are going to be critical moving forward, especially when large-scale spectroscopic surveys, such as the Dark Energy Spectroscopic Instrument, the Vera C. Rubin Observatory and Euclid, start releasing the vast amounts of data they are collecting of the sky.
“Soon we’re going to have a petabyte-scale data flow,” Ho explained. “That’s huge. It’s impossible for humans to parse that by hand. As we work on building models that can be robust estimators of things like mass while mitigating sources of error, another important aspect is that they need to be computationally efficient if we’re going to process this huge data flow from these new surveys. And that is exactly what we are trying to address — using machine learning to improve our analyses and make them faster.”
This work is supported by the NSF and the McWilliams-PSC Seed Grant Program. The computing resources necessary to complete this analysis were provided by the Pittsburgh Supercomputing Center.
The study’s authors include: Hy Trac, an associate professor of physics; Michelle Ntampaka, who graduated from CMU with a doctorate in physics in 2017 and is now deputy head of Data Science at the Space Telescope Science Institute; Markus Michael Rau, a McWilliams postdoctoral fellow who is now a postdoctoral fellow at Argonne National Lab; Minghan Chen, who graduated with a bachelor’s degree in physics in 2018, and is a Ph.D. student at the University of California, Santa Barbara; Alexa Lansberry, who graduated with a bachelor’s degree in physics in 2020; and Faith Ruehle, who graduated with a bachelor’s degree in physics in 2021.
■ Amy Pavlak Laird
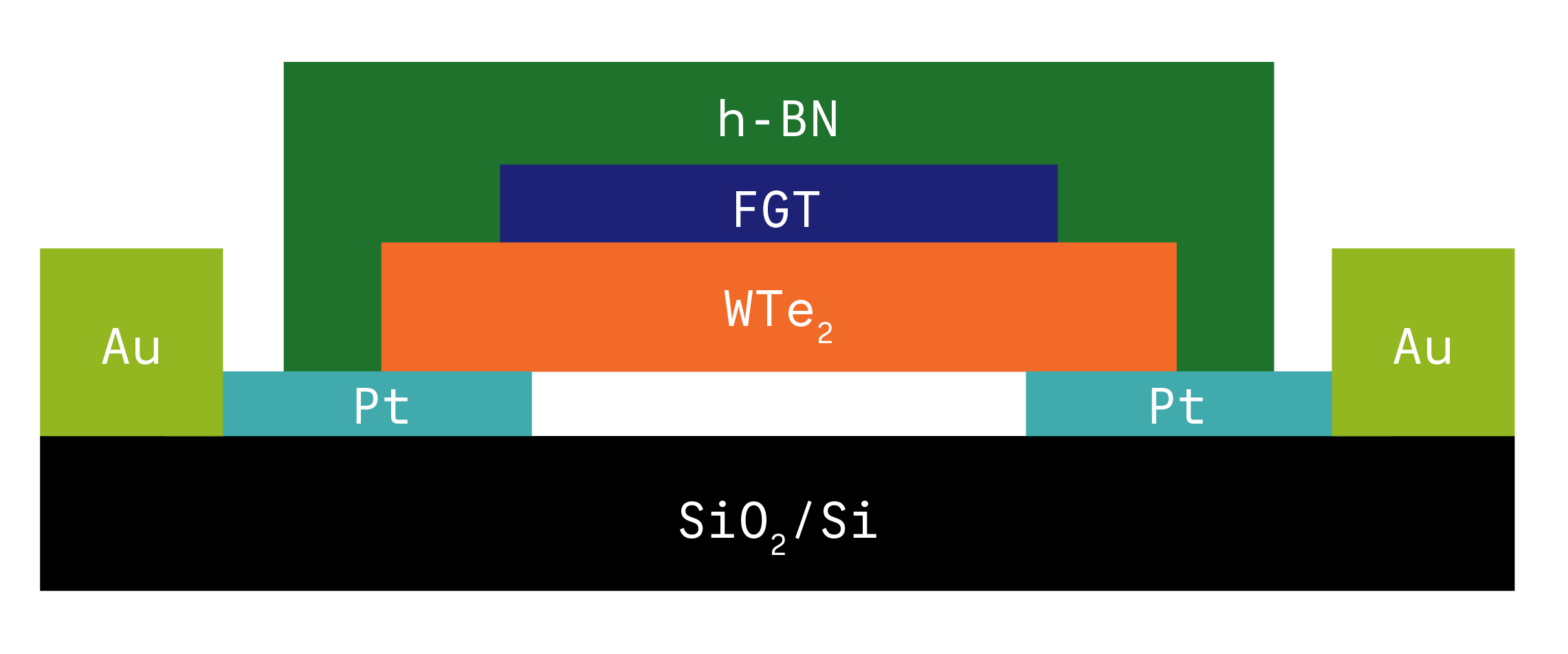
Physicists Switch Magnetic State Using Spin Current
When Carnegie Mellon University doctoral candidates I-Hsuan Kao and Ryan Muzzio started working together a switch flicked on. Then off.
Working in the Department of Physics’ Lab for Investigating Quantum Materials, Interfaces and Devices (LIQUID) Group Kao and Muzzio and other research partners were able to show proof of concept that running an electrical current through a novel two-dimensional material could control the magnetic state of a neighboring magnetic material without the need of applying an external magnetic field.
The groundbreaking work, which was published in Nature Materials in June and has a related patent pending, has potential applications for data storage in consumer products such as digital cameras, smartphones and laptops.
“What we’re doing here is utilizing ultrathin materials — often the thickness of few atoms — and stacking them on top of each other to create high-quality devices,” said Kao, who was first author on the paper.
Simranjeet Singh, an assistant professor of physics and Jyoti Katoch, an assistant professor of physics, oversee the LIQUID Group, which investigates the intrinsic physical properties of two-dimensional quantum materials such as tungsten ditelluride (WTe2) and their electronic and spin-related properties.
“Spins and magnetism are everywhere around us,” Singh said. “Atoms configure in a particular way on an atomic lattice that in turn dictates material properties. For WTe2, it has a low-symmetry crystal structure that allows us to generate a special kind of spin current by applying an electric field.”
The way atoms are configured in WTe2 allows for an out-of-plane oriented spin current that in turn can be used to control the magnetization state of a magnet. Singh said that in order to switch the magnetic state (up or down) of most magnetic materials using spin current studied so far, a magnetic field is applied horizontally, or in plane. Having a material that can switch magnetism without the need of an external magnetic field can lead to energy-efficient data storage and logic devices.
The work could be applied to magnetoresistive random-access memory (MRAM) devices, which have the potential of realizing high-speed and densely packed data storage bits while using less power.
“People can do this already, you can take a material, apply an electric field to generate in-plane oriented spin current and use it to switch the magnetization from an up state to a down state or vice-versa, but it requires an external magnetic field,” Muzzio said. “What this boils down to is finding a material that has the intrinsic property that includes breaking symmetry.”
Kao brought expertise on magnetism, while Muzzio understood how to build the devices as well as studies the behavior of electrons in material systems. To show that the behavior was reproducible, Kao and Muzzio created more than 20 devices over two years.
The simple devices are miniscule and allow a switch to either be turned to an up position or a down position. “Think of it like zeros and ones in binary,” Kao said. While the devices could be 3-50 microns in length or width, the thickness is smaller than 1/200th of a human hair.
“We’ve just scratched the surface of what this material can do,” Muzzio said. “There’s so much more parameter space for us to explore and so many ways to utilize this material. This is just the beginning.”
In addition to Kao, Muzzio, Singh and Katoch, co-authors on the paper “Deterministic switching of a perpendicularly polarized magnet using unconventional spin-orbit torques in WTe2” include Hantao Zhang and Ran Cheng of the University of California Riverside; Menglin Zhu and Joshua E. Goldberger of The Ohio State University; Daniel Weber of The Ohio State University and the Karlsruhe Institute of Technology, Germany; Jacob Gobbo, who graduated from CMU in 2020 and is a doctoral candidate at the University of California, Berkley; Sean Yan, a rising senior in physics at CMU; Rahul Rao of the Air Force Research Laboratory at Wright-Patterson Air Force Base; Jiahan li and James H. Edgar, both of Kansas State University; and Jiaqiang Yan of Oak Ridge National Laboratory and The University of Tennessee.
Funding for this research was provided primarily by the Center for Emergent Materials — a National Science Foundation Materials Research Science and Engineering Center at The Ohio State University, the Air Force Office of Scientific Research, the U.S. Department of Energy, the Gordon and Betty Moore Foundation, the German Science Foundation and the Office of Naval Research.
■ Heidi Opdyke
The Strange Behavior of Sound Through Solids
Not everything needs to be seen to be believed; certain things are more readily heard, like a train approaching its station. In a recent paper, published in Physical Review Letters, researchers have put their ears to the rail, discovering a new property of scattering amplitudes based on their study of sound waves through solid matter.
Be it light or sound, physicists consider the likelihood of particle interactions in terms of probability curves or scattering amplitudes. It is common lore that, when the momentum or energy of one of the scattered particles goes to zero, scattering amplitudes should always scale with integer powers of momentum (i.e., p1, p2, p3, etc.). What the research team found however, was that the amplitude can be proportional to a fractional power (i.e., p1/2, p1/3, p1/4, etc.).
Why does this matter? While quantum field theories, such as the Standard Model, allow researchers to make predictions about particle interactions with extreme accuracy, it is still possible to improve upon current foundations of fundamental physics. When a new behavior is demonstrated — such as fractional-power scaling — scientists are given an opportunity to revisit or revise existing theories.
This work, conducted by The Institute for Advanced Study’s Angelo Esposito, The University of Stavanger’s Tomáš Brauner, and Carnegie Mellon’s Riccardo Penco, specifically considers the interactions of sound waves in solids. To visualize this concept, picture a block of wood with speakers placed on both ends. Once the speakers are powered on, two sound waves — phonons — meet each other and scatter, similar to collisions in a particle accelerator. When one speaker is adjusted to a certain limit, such that the momentum of the phonon is zero, the resulting amplitude can be proportional to a fractional power. This scaling behavior, the team explains, is not likely limited to phonons in solids, and its recognition may help the study of scattering amplitudes in many different contexts, from particle physics to cosmology.
“The detailed properties of scattering amplitudes have recently been studied with much vigor,” Esposito said. “The goal of this broad program is to classify possible patterns of behavior of scattering amplitudes, to both make some of our computations more efficient, and more ambitiously, to build new foundations of quantum field theory.”
Feynman diagrams have long been an indispensable tool of particle physicists, yet they come with certain limitations. For example, high accuracy calculations can require tens-of-thousands of Feynman diagrams to be entered into a computer, to describe particle interactions. By gaining a better understanding of scattering amplitudes, researchers may be able to more easily pinpoint particle behavior rather than relying on the top-down approach of Feynman diagrams, thus enhancing the efficiency of calculations.
“The present work reveals a twist in the story, showing that condensed matter physics displays much richer phenomenology of scattering amplitudes than what was previously seen in fundamental, relativistic physics,” Esposito said. “The discovery of fractional-power scaling invites further work on scattering amplitudes of collective oscillations of matter, placing solids in the focus.”
■ Lee Sandberg
neutrino’s mass smaller than previously known
The neutrino, the tiniest of the fundamental particles, is even smaller than previously known. The Karlsruhe Tritium Neutrino Experiment (KATRIN), an international team of researchers including Carnegie Mellon physicist Diana Parno, has established a new upper limit on the neutrino’s mass at less than 0.8 electron volts (eV). The team’s findings were published in Nature Physics.
The weight of the neutrino is an important missing piece of Standard Model of particle physics. A theory that describes the fundamental forces of the universe and the known elementary particles, the Standard Model has been used to explain many phenomena seen in the universe and in particle physics. But the Standard Model as it was written some 50 years ago isn’t perfect. One flaw is that it assumed that neutrinos were massless.
While neutrinos may be extremely light — more than a half a million times lighter than the electron — there are an astounding number of neutrinos in the universe.
“Just like a pound of lead and a pound of feathers take up a different amount of space, their mass is still the same,” said Parno, assistant professor in Carnegie Mellon’s Department of Physics. “Neutrino mass plays an important role in the universe’s expansion and the formation of structures, like galaxy clusters. Knowing the mass of the neutrino is essential to understanding our universe.”
Detecting and weighing the neutrino is a difficult task. Neutrinos have no charge and they rarely interact with other matter.
KATRIN, located at Germany’s Karlsruhe Institute of Technology, measures neutrino mass using the beta decay of tritium, which emits a pair of particles: one electron and one neutrino. The researchers measure the electrons’ energy and then use that to calculate the energy and mass of the neutrino.
The KATRIN experiment will continue to collect data for several years. The researchers hope that they can further narrow the parameters for the neutrino’s mass and continue to improve the Standard Model.
The KATRIN collaboration involves scientists from six countries and is funded by a number of international entities. The U.S. efforts are supported by the Department of Energy (DOE) Office of Nuclear Physics.
Funding for this research was provided primarily by the Center for Emergent Materials — a National Science Foundation Materials Research Science and Engineering Center at The Ohio State University, the Air Force Office of Scientific Research, the U.S. Department of Energy, the Gordon and Betty Moore Foundation, the German Science Foundation and the Office of Naval Research.
■ Jocelyn Duffy