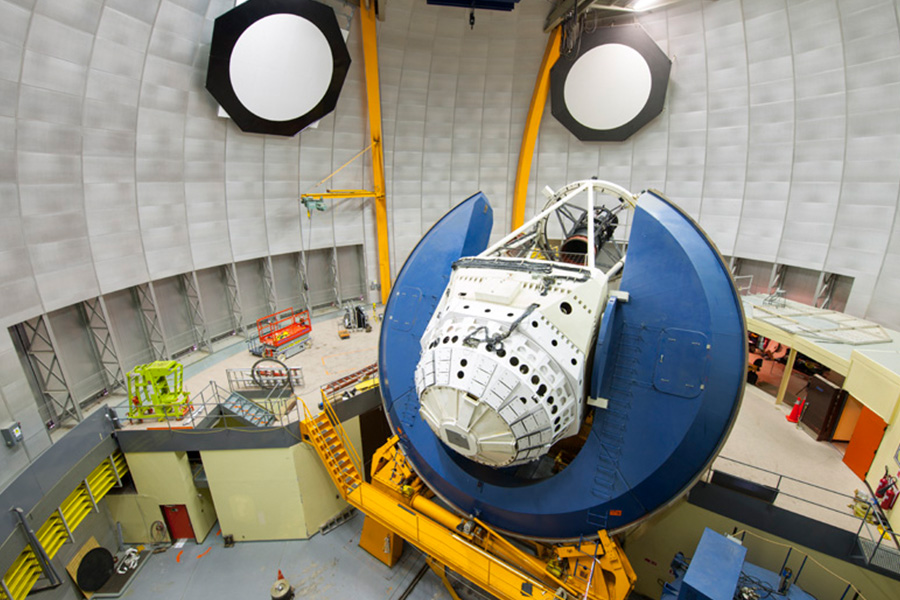
The Dark Energy Survey photographed the night sky using the 570-megapixel Dark Energy Camera on the 4-meter Blanco telescope at the Cerro Tololo Inter-American Observatory in Chile, a Program of the National Science Foundation’s NOIRLab. Reidar Hahn/Fermilab
ai
in physics
Planning for the Future
From the billions of galaxies in the observable universe that will be imaged by next-generation telescopes, to the 90 petabytes of data produced each year by the Large Hadron Collider, to modeling the millions of proteins in a cell, physics research has become a big data enterprise. One of the biggest challenges for today’s scientists is creating ways to manage and analyze the vast amounts of data that are produced and collected by modern physics experiments.
Last summer, the National Science Foundation (NSF) awarded Carnegie Mellon University a $500,000 grant to prepare to take on this challenge by creating the Planning Institute for Data-Driven Discovery in Physics, which supports the university as it builds the capacity to become a full institute. This project is part of the NSF’s National Artificial Intelligence Research Institutes program which is the agency’s most significant investment in AI research and workforce development to-date. The institutes, which are in a wide range of fields, focus on advancing foundational artificial intelligence (AI) research, accelerating innovation in scientific fields using AI, building the next generation of talent and bringing together scientists from a wide range of fields for interdisciplinary collaboration.
Creation of the planning institute allowed Carnegie Mellon and the Department of Physics to capitalize on their existing strengths in physics, data science, AI and collaborative research. Since announcing the institute in late August 2020, the Department of Physics and its partners have made significant advances in AI-driven physics research and created new venues for collaboration and education.
Accelerating Innovation
Research being done by Carnegie Mellon physicists illustrates how the university’s unique combined talents in physics, AI and data science fuels the collaborations that are accelerating science.
Physics Professors Tiziana Di Matteo and Rupert Croft and Ph.D. candidate Yueying Ni, with collaborators from the Flat Iron Institute, University of California, Riverside and University of California, Berkeley brought together machine learning, high-performance computing and astrophysics to create a complex simulated universe in less than a day.
Cosmological simulations, like those created by Di Matteo and Croft’s research groups, are an essential part of teasing out the many mysteries of the universe, including those of dark matter and dark energy. But, due to computational constraints, researchers could only focus on a small area at high resolution or a large volume at low resolution in their simulations. In the new work, published in the Proceedings of the National Academy of Sciences, the researchers surmounted this problem by teaching a machine learning algorithm based on neural networks to upgrade a low-resolution simulation to super resolution.
“With our previous simulations, we showed that we could simulate the universe to discover new and interesting physics, but only at small or low-res scales,” said Croft. “By incorporating machine learning, the technology is able to catch up with our ideas.”
Carnegie Mellon researchers also played a pivotal role in the Dark Energy’s Survey’s (DES’s) recent creation of the largest and most precise maps of the distribution of galaxies in the universe at relatively recent epochs. The university’s expertise in weak gravitational lensing, artificial intelligence and data science were key to the DES analyses.
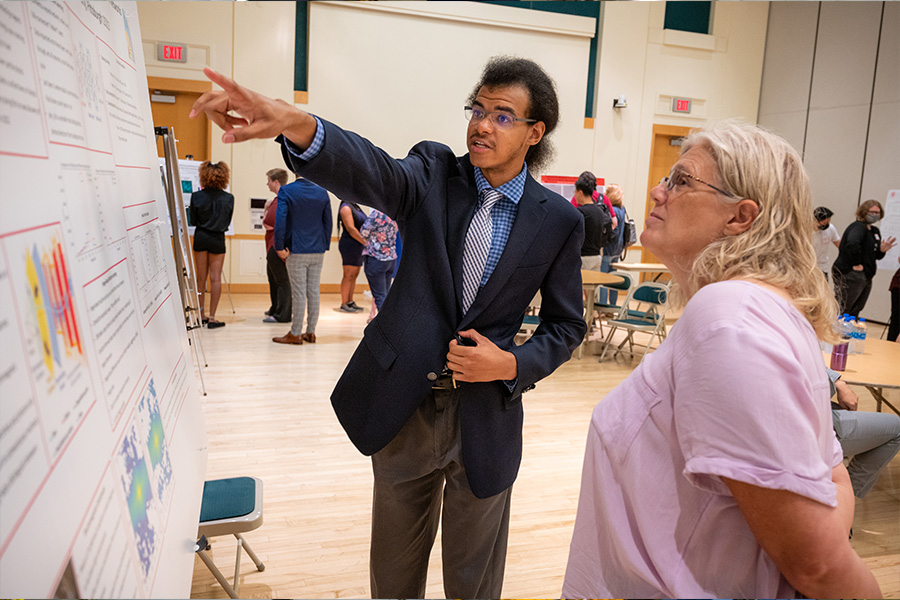
Jonah Warner (Penn State University) participates in the poster session as part of the AI Planning Institute Artificial Intelligence and Physics Summer Undergraduate Research Program
Postdoc Simon Samuroff co-led the weak gravitational lensing analysis, using a variety of artificial intelligence tools to calibrate the redshift distribution of galaxy samples. Ph.D. candidate Andresa Campos ran Markov Chain Monte Carlo analyses to extract constraints on parameters for measuring galaxy clusters and supernovae.
“We used over 30 parameters to fit this complex data set,” said Campos. Campos and Dodelson also worked within DES to develop tools to assess consistency between DES and Planck data in these highly multi-dimensional spaces. “We used to be able to simply look at two measurements of a single number and tell instantly whether they were consistent. Now, in these multi-dimensional parameter spaces, we need the full power of Bayesian statistics and modern data science.”
“One of the greatest discoveries we have made in this round is that the statistical power of DES and future data sets will require a new set of tools borrowed from, and developed in conjunction with, the data science community,” said Dodelson.
Creating Opportunities for Interdisciplinary Collaboration
Through the institute, the Department of Physics has been able to bring together researchers working at the interface of physics and artificial intelligence for virtual lectures, conferences and informal discussions.
Hundreds of physicists and data scientists participated in “Quarks to Cosmos with AI,” a virtual workshop held this summer. As hosts of the workshop, Carnegie Mellon was able to showcase its expertise in AI, machine learning, data science and physics while learning from colleagues. The conference was co-organized by Physics Professors Tiziana Di Matteo, Rachel Mandelbaum and Manfred Paulini, Statistics and Data Science Assistant Professor Mikael Kuusela and Professor Ann Lee. Over the course of a week, more than 200 academics, industry professionals and students from around the world logged in to hear a variety of lectures from leading researchers in physics and machine learning.
A highlight of the workshop was daily hackathons using datasets and computing resources provided by the Pittsburgh Supercomputing Center. These events allowed scientists at a variety of stages in their careers to collaborate in analyzing and solving data challenges in a diverse range of fields, including cosmology and particle physics.
“For me, the most important result of the hackathon was the engagement of the students and young researchers, who had the opportunity to learn about modern machine learning and AI environments and play with them on state-of-the-art computing facilities provided by the Pittsburgh Supercomputing Center,” Paulini said.
Last year, the institute hosted the virtual “AI-Driven Discovery in Biophysics” conference, organized by Assistant Professor of Physics Shila Banerjee and Professor of Computational Biology Russell Schwartz. The conference featured speakers from Carnegie Mellon and the University of Pittsburgh working in computational biology, mechanical engineering and chemistry, a poster session and a panel discussion about the future of AI and biophysics.
Weekly, the institute hosts a virtual seminar featuring speakers from organizations around the world, as well as from the university, to talk about their work. It also partners with STAMPS@CMU (Statistical Methods for the Physical Sciences) for virtual webinars and has hosted informal coffee hours to promote interaction between researchers.
Building the Next Generation of Talent
As part of its educational mission, the AI Planning Institute hosted its first Artificial Intelligence and Physics Summer Undergraduate Research Program in 2021 and is bringing the program back for 2022. The eight-week, fully funded opportunity allows undergraduate students to explore the intersection of AI and physics. Students are mentored by a team that consists of a faculty member, postdoc and graduate student.
Students came to Carnegie Mellon to participate in the program from Morehouse College, Penn State University and Carnegie Mellon. They worked on projects in astrophysics and particle physics and presented their research at a poster session alongside students who participated in other summer programs sponsored by the Mellon College of Science.
Also over the summer, John Urbanic, a parallel computing specialist at the Pittsburgh Supercomputing Center and visiting researcher in the Department of Physics, brought AI to the high school students participating in the Pennsylvania Governor’s School for the Sciences. The course called “A Working Introduction to AI” covered the kinds of data science and machine learning increasingly impacting the sciences including a hands-on introduction to the tools used to do large scale data analytics and for using neural nets. The students embraced the materials enthusiastically and many already had notions of using them in projects, Urbanic said.
Looking To the Future
The planning institute grant runs through summer 2022, and the university will then be considered for funding for a full institute. No matter the outcome, the Department of Physics will continue their work integrating physics and AI. This summer, Carnegie Mellon announced the launch of a future of science initiative that will support research of the future, specifically research that brings together AI, machine learning and the foundational sciences.
■ Jocelyn Duffy